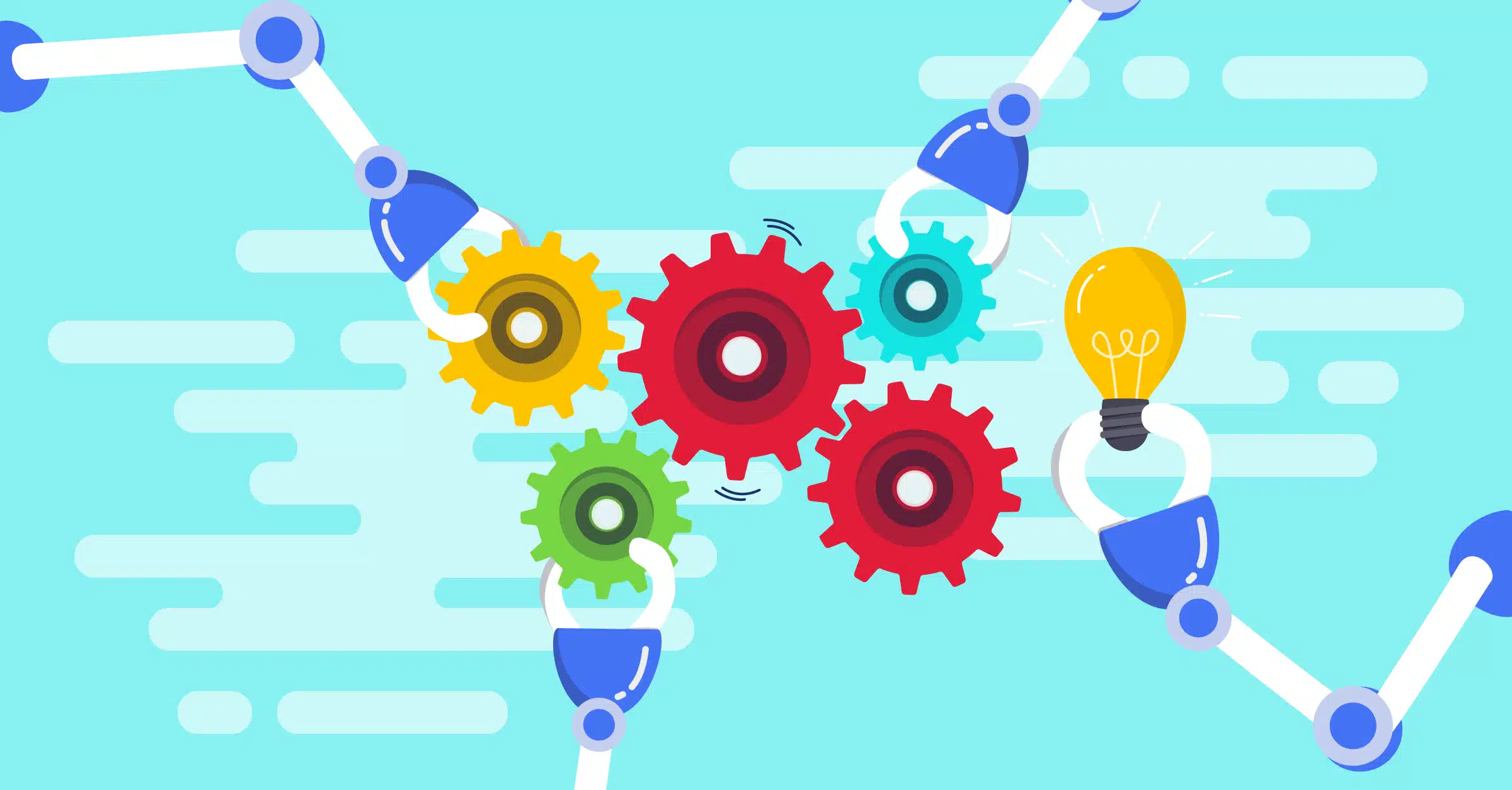
Understanding AI’s True Capabilities—Practical Applications and Key Considerations for Organizations
Artificial intelligence (AI) has made a significant impact across industries, forcing organizations to quickly consider what it means for their future and how they can use it. While AI holds incredible potential to change the world, we are still in the early stages of fully understanding its capabilities. Currently, there’s a lot of misconception about what AI can do, and many conversations around it are overly optimistic.
Take the example of doing laundry. Some discussions around AI paint a picture where AI not only sets the temperature but also puts the clothes in the washing machine, pours detergent, takes them out, folds them, and puts them away. The reality is much simpler—AI today can set the temperature, but that’s about it. While the ability to automate a task like setting the temperature is valuable, we are still far from the scenario where AI can manage the entire laundry process.
The key takeaway is that AI, for now, should be seen as a tool that can automate specific components of a process, not the entire process. As AI continues to evolve, it’s important for organizations to assess their business processes and determine which tasks are suitable for AI implementation. To do this, consider the following features when evaluating potential use cases:
Characteristics of Tasks for AI Automation
It’s important to distinguish between AI and traditional automation. While both involve data input to guide a machine’s actions, automation simply repeats the same action it’s been programmed to do, regardless of errors. AI, on the other hand, uses real-time data to recognize errors and adjust its actions accordingly.
Data Availability
AI models require large, structured datasets to be effective. These models perform best when they have consistent and reliable data to learn from, such as databases and spreadsheets.
Consistency of Process
Tasks that are repetitive, predictable, and well-defined, like sorting, scheduling, or classifying, are ideal for automation with AI.
Speed
AI can support tasks that require faster decision-making where speed is crucial.
Scalability
If a task is expected to increase in volume over time, AI can scale effectively to handle the increased demand.
Error-Prone
Tasks with a high probability of human error can benefit from AI, reducing the likelihood of mistakes.
Skills
Tasks that require human labor but don’t demand specific technical skills are well-suited for AI automation.
Frequency
Tasks that occur frequently are ideal candidates for AI implementation to improve efficiency.
Understanding AI Techniques
AI uses different techniques to address various types of tasks.
Machine Learning (ML)
ML algorithms learn from data and improve over time without needing explicit programming. These models are particularly useful in scenarios that require predictive analysis.
Deep Learning
A subset of ML, deep learning attempts to simulate the human brain’s ability to process data. It’s particularly effective for tasks like speech and image recognition.
Natural Language Processing (NLP)
NLP enables AI to understand and generate human language in a meaningful way. Chatbots are a prime example of this application.
Generative AI: The Next Frontier
Generative AI, powered by large language models, is another emerging area worth understanding. These models are trained on vast amounts of data to learn patterns and relationships, allowing them to generate content that often mimics human creations. While this has exciting applications, it also presents unique challenges in terms of data security and intellectual property.
My AI Journey: Contract Redlining for Research Institutions
To illustrate the practical challenges and opportunities of AI, I’ll share my experience using AI for contract redlining. My team negotiates research-related contracts, which come in countless varieties. In fact, 50% of the time we spend on these contracts is used to produce the initial redline. The problem seemed ripe for AI intervention.
The Problem
Our goal was to reduce the time spent generating redlines by using AI. We assumed that with enough precedent contracts, we could train an AI model to automate this process. The model would eventually speed up our contract review process, making it more efficient.
The Reality
However, our assumptions didn’t hold up. We tried piloting four AI solutions, all built on large language models. While they showed immense potential, we encountered several challenges.
1. Clean Data
Challenge: The primary challenge was ensuring high-quality, standardized data for training the AI model. Research contracts often use varied language, with different negotiators expressing similar outcomes in distinct ways.
Lesson Learned: To build an effective AI model, it was crucial to clean, structure, and normalize the data to ensure consistency and accuracy during training.
- Data quality over quantity. More data isn’t always better. Focus on collecting high-quality, relevant data rather than large volumes of inconsistent information.
- Standardization is key. Consistent data formatting and labeling prevent confusion for AI models and improve output accuracy.
- Invest in data profiling. Use data-profiling tools to identify patterns, outliers, and errors before feeding the data into your AI model.
- Collaborate with subject matter experts. Work closely with domain experts to ensure the cleaned data aligns with business needs and nuances.
- Document your data cleanup process. Maintaining clear documentation helps with future model retraining and knowledge transfer.
- Automate where possible. Use extract, transform, and load (ETL) tools or data cleansing platforms to automate repetitive tasks, reducing human error and accelerating the process.
- Plan for ongoing maintenance. Data cleanup isn’t a one-time task. Establish continuous monitoring to maintain data integrity as new information flows in.
2. Subject Matter Expertise
Challenge: To effectively implement an AI solution you need both a subject matter expert in the technology involved as well as a subject matter expert in the type of work you are trying to improve with the technology.
Lesson Learned: Ensure you create adequate time for your subject matter experts to collaborate to implement to the solution effectively.
3. Adoption Challenges
Challenge: AI models are most adept at flagging problematic language. While this was useful for junior staff, more experienced staff found it to be a hindrance. Their methods for spotting issues were faster than the AI’s suggestions.
Lesson Learned: As with any new system, a detailed rollout plan and staff training are crucial. It took time for the team to see the value of the AI system, and its benefits were more apparent as the model learned over time.
4. Data Privacy and Security
Challenge: A final consideration when selecting an AI vendor is understanding how your data will be used. Some platforms may use your data to train their broader model, which can be a concern if you’re working with sensitive information.
Lesson Learned: Always review the terms and conditions carefully and ensure that your data remains protected.
Attain Partners – Helping Your Organization Leverage AI for Impact
From reviewing terms of use to ensuring clean data, training the model, and navigating adoption challenges, selecting and implementing AI is not a straightforward process. It’s critical to approach AI implementation with realistic expectations, adequate planning, and ongoing fine-tuning to achieve the desired results.
At Attain Partners, we understand the complexities involved in choosing and implementing AI solutions. Our goal is to help our clients identify and implement the AI technologies that are right for their organizations, ensuring that the benefits far outweigh the challenges. By carefully considering factors like data quality, training, and adoption strategies, organizations can unlock the true potential of AI and drive lasting improvements in their operations.
As AI continues to evolve, we remain excited about its potential to reshape industries, but we also recognize that its true power lies in understanding its current limitations and approaching its integration thoughtfully.
If your organization needs help selecting AI solutions, implementing AI solutions, or cleaning data for AI solutions, contact our experts, Istvan Fekete or Ryan Hartley.
About the Authors
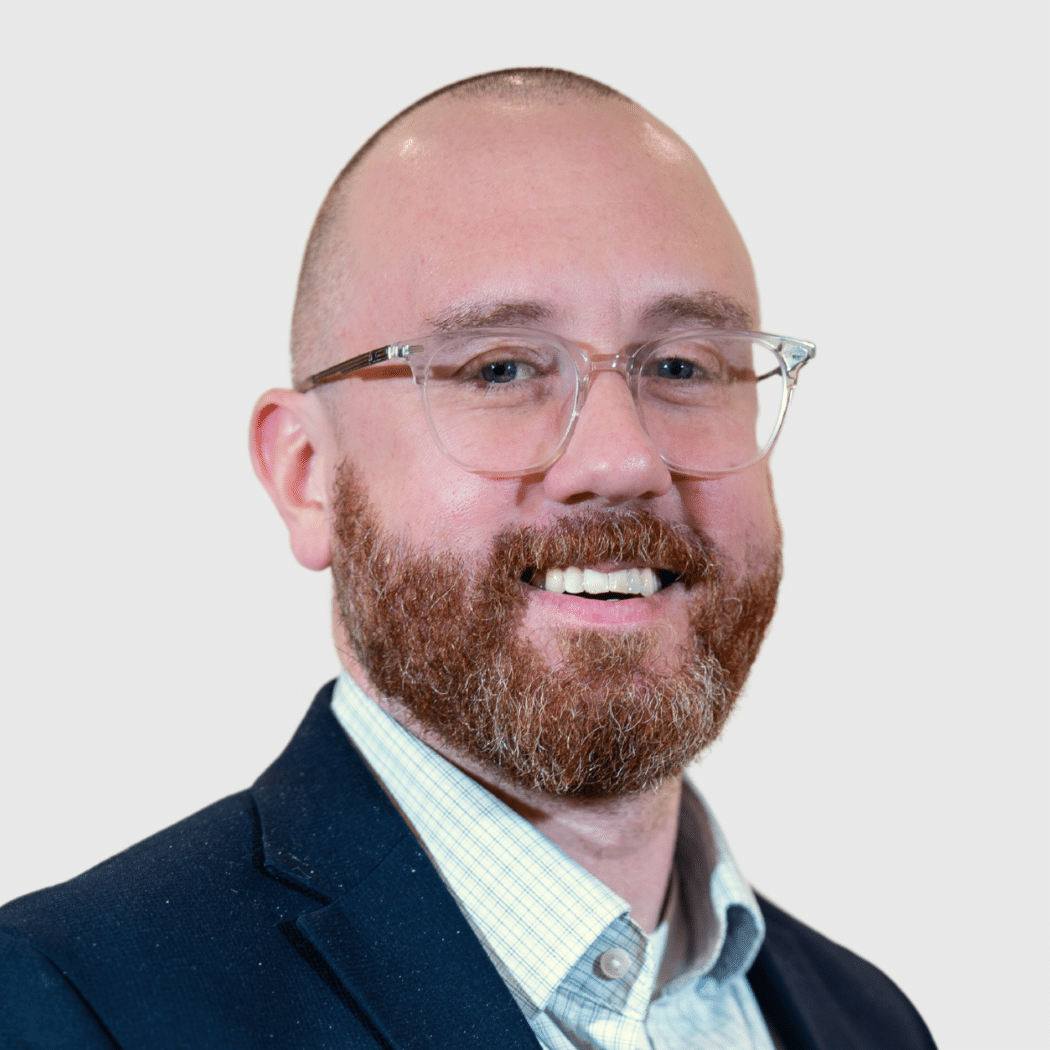
Istvan Fekete is a Principal at Attain Partners, specializing in clinical research, contracting, and artificial intelligence. With over 13 years of progressive research administration experience, he previously served as Assistant Director of Contracts at the University of Chicago, where he developed numerous systems and processes to increase contract processing efficiency and decrease contract turnaround times. Istvan has worked with over 50 organizations, including universities, hospitals, academic medical centers, non-profits, and pharmaceutical companies. He has published articles in NCURA Magazine on topics such as the use of biological samples in clinical research and research administration in a virtual world. Istvan holds a Bachelor of Business Administration in Management, magna cum laude, from Loyola University Chicago.
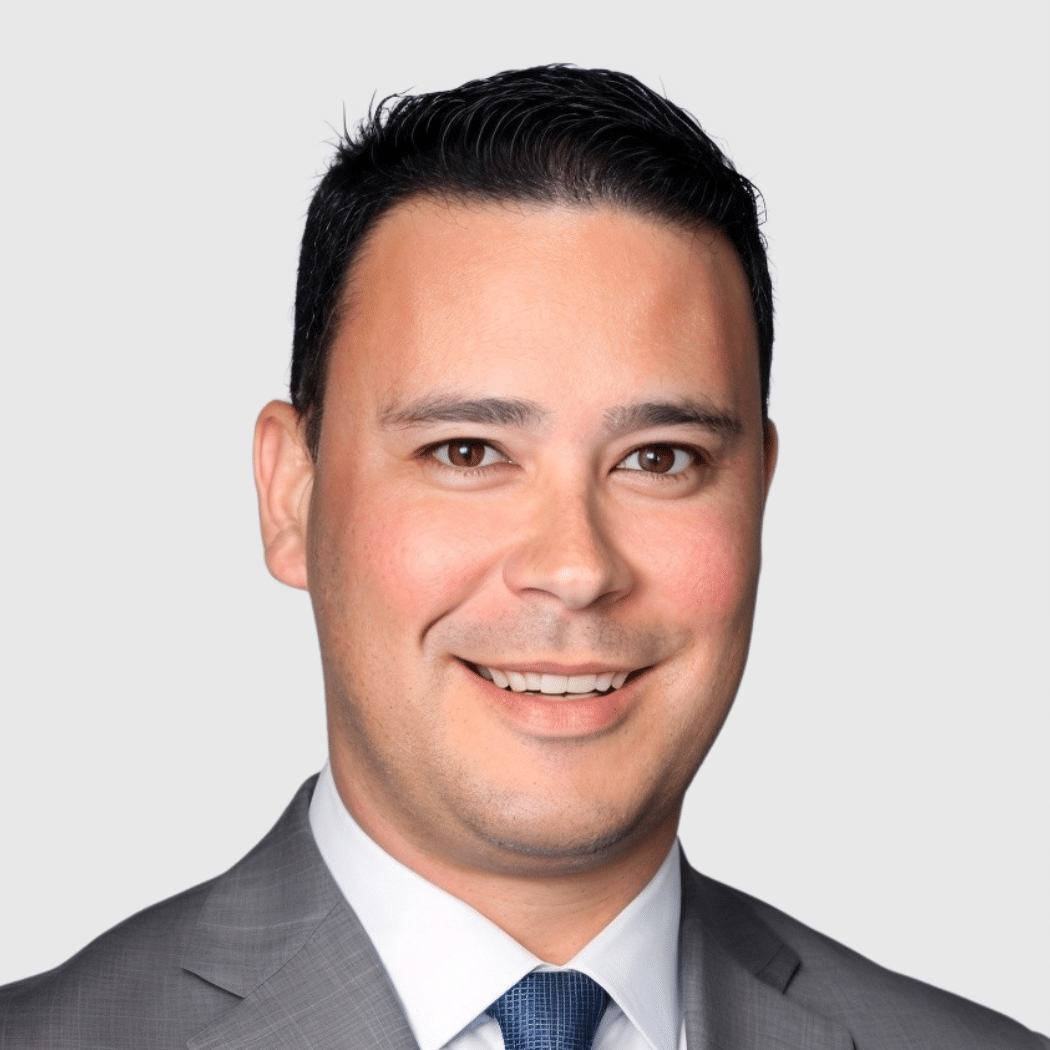
Ryan Hartley is a Managing Director at Attain Partners, where he leads Data Services within the Attain Digital practice, delivering innovative and scalable solutions to help clients unlock the full potential of their data for operational excellence and strategic growth. With over 15 years of experience in data integration, master data management (MDM), data quality, governance, and advisory services, Ryan has a proven track record of transforming data into a strategic asset across diverse industries, including higher education, nonprofit, healthcare, retail, and manufacturing. Prior to joining Attain Partners, he held leadership roles overseeing large-scale technology initiatives focused on customer relationship management (CRM), MDM, and data governance. Ryan is known for his innovative approach, collaborative leadership, and ability to align technology strategies with business objectives to deliver measurable results.