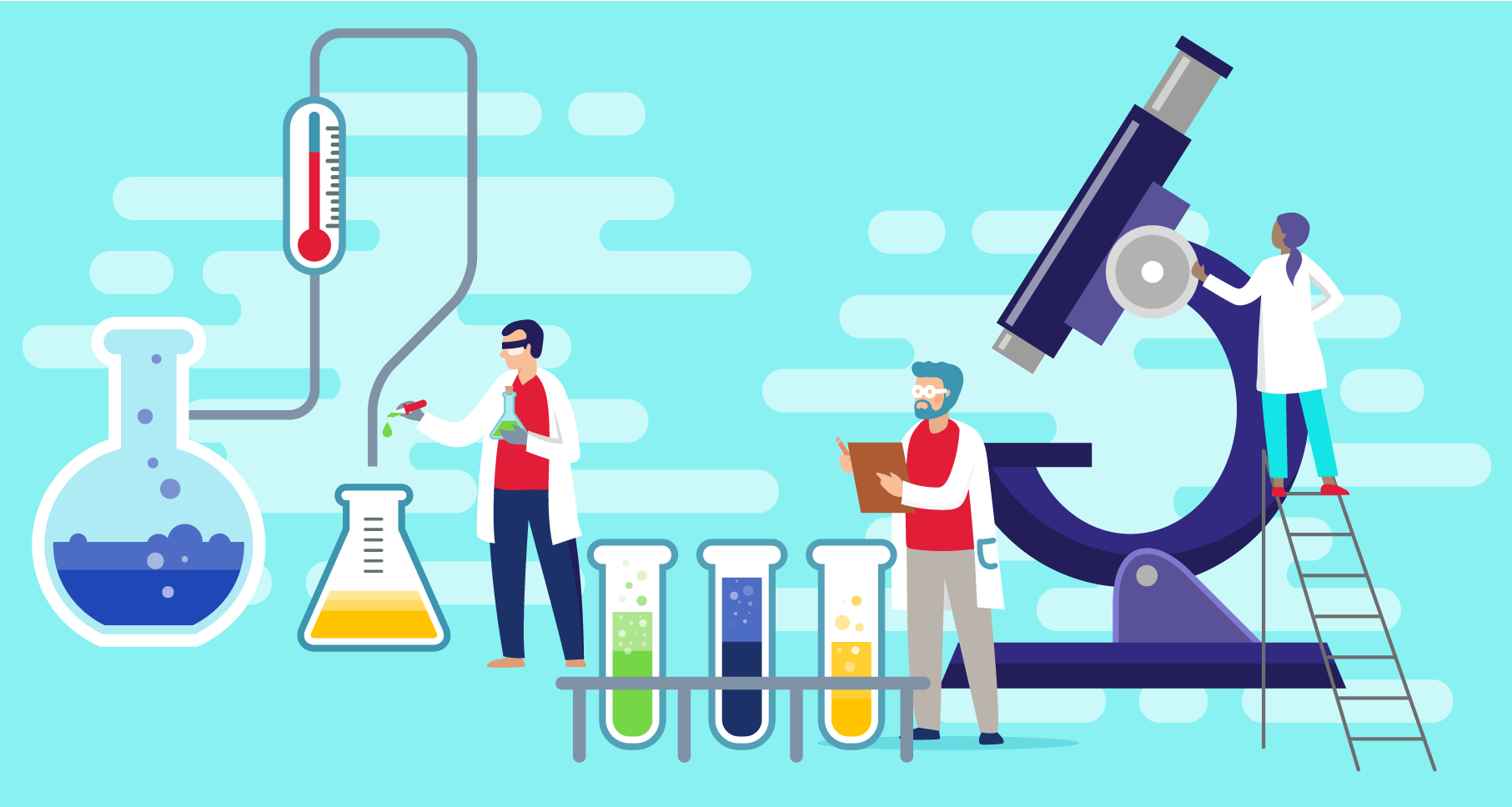
Introduction
In this post, we will cover the five basic steps to calculate a research density metric. Specifically, this research density metric will use expense as a proxy for research output. If this sounds confusing, check out the first post in our research density series which explains research density metrics.
Before getting into the five steps, it’s important to remember that like any approach or methodology, there are many ways and rationales for calculating research density. Our goal here is to discuss the approach we believe has both the highest likelihood of success and the lowest ongoing cost of execution.
Step 1: Select a unit and period of analysis
One consideration in any analysis is the level or levels at which the analysis will be conducted. In most cases, this consideration is a battle between specificity and accuracy. Specificity refers to the deepness/smallness of the unit of analysis. For example, the least specific unit will generally be the institution as a whole and the most specific unit for most institutions is the principal investigators (PIs) or even, for a few institutions, the projects. Accuracy refers to how well an analysis represents reality. In most cases, the level of accuracy is derived from the quality of data. In some cases, the accuracy of an analysis is marred by errors in the analysis rather than poor-quality data, but that can be avoided by using standardized software or mitigated by carefully reviewing and validating custom analyses. In almost every case, accuracy is preferred over specificity.
For example, accurate metrics at the college level are generally more valuable than inaccurate metrics at the PI level. While this may sound obvious, it is not uncommon for organizations to conduct analyses at a desired specificity without considering the impact on accuracy. For this reason, we generally recommend institutions conduct analyses at the lowest level of units that is reliably tracked in their systems of records. For example, if an institution reliably attributes space to departments but does not reliably attribute space to PIs, we recommend conducting analyses that utilize space, such as research density, at the department level rather than the PI level.
Once you’ve assessed the lowest level at which an accurate analysis can be created, most often the lowest level and above will be selected. So, if an analysis can be done at the department level, then the levels of analysis will be department, division, and institution. For the rest of this article, we will track an illustrative example to help explain each step.
Illustrative Example
- Level(s) of Analysis: departmental level
- Sample from Level: Chemistry
If you are conducting this analysis for the first time and doing so manually, in place of a purpose-built tool such as Attain Research Performance (Attain RP), we encourage starting with a single, full year of data in which you are confident in both the accuracy and completeness of the dataset.
Illustrative Example
- Year of Analysis: 2020
Step 2: Pick a specific metric or metrics for analysis
Other articles in this series will cover this decision in more detail, but we often strongly encourage institutions to start with indirect cost recovery density. There is an array of reasons for this, but the most straightforward is that leadership tends to quickly understand the value of improving this metric.
Illustrative Example
- Metric for Analysis: IDC Recovery Density
Having said that, the process outlined here will apply to any research density metric you might use. The core consideration here is the availability of accurate and complete data for the research density metrics that you want to create.
Step 3: Sum expense items for the metric
There are several options here depending on your level of expertise in Excel or other analysis tools. The two general approaches are bottom-up calculations or exported rollups. Bottom-up calculations are those in which atomic line-item data are exported from the systems of records and are rolled up in the analysis, while exported rollups are, as the name implies, where the systems of records do the rolling up internally and then simply exports the aggregated information.
Given the authors’ backgrounds, we both strongly prefer bottom-up calculations, which place the burden or opportunity of parsing, filtering, and rolling up the relevant transactions on the analyst. This preference comes from the ability to validate, refine, or extend the analysis, which is generally not as feasible with exported rollups. The tradeoff is the additional bandwidth and/or expertise required as compared to exported rollups.
It is worth noting that the feasibility of exported rollups does depend on the features of the systems of records. However, most ERP systems provide rollups, at least based on organizational units. By leveraging this feature, the summation of financial data can be done very quickly if the level desired is available for rollup with the transaction type selected.
Illustrative Example
- Bottom-up: Sum all IDC Recovery transactions for Chemistry in 2020 for a value of $2,000,000
Once this step has been completed, you will have the numerator for your density analysis and now just need to calculate the denominator.
Step 4: Calculate the ASF in the period of analysis
The availability and accuracy of the space data often determines the level of analysis for a given period, but once that has been determined the next steps are relatively straightforward. Assignable Square Feet (ASF) represents the space that is used or available for use by the institution. As such, it is calculated by simply taking the total area assigned to a given unit and reducing that by the vacant and unassignable space assigned to that unit.
Illustrative Example
- ASF: Sum total area for Chemistry in 2020 and subtract the unassignable and vacant space | ASF 11,500 = Total Area 15,000 – (VAC 2,500 + UN 1,000)
Step 5: Divide the expense value by the ASF value
At this point, the hard work is done, and you simply divide the expense metric(s) from Step 3 by the ASF from Step 4 for each unit at each level of analysis.
Illustrative Example
- IDC Recovery Density: IDC Recovery 173.91 / ASF 11,500
A note on execution
The goal of this article is to express the simplicity of the theory behind computing a density metric We recognize that even with resolutions to the data accuracy and specificity challenges, there are various other challenges in building and maintaining reliable analyses. With that being said, the most important thing we recommend for institutions, when it comes to metrics, is that they start tracking at least some of them as soon as possible. Even if it means tracking just one or a few, establishing a system of data-driven monitoring and decision making is key to running and growing a successful research enterprise.
For institutions with the willingness, ability, and interest in spending the time and resources to conduct the analyses themselves, we absolutely encourage getting started or incorporating research density into existing analyses. In fact, we, the authors, are personally happy to meet with you and give some tips on getting started because we believe every institution should and would benefit from tracking their metrics, particularly research density. For those not interested in the challenge of running these analyses, we especially recommend contacting us below to find out about how our product, Attain RP, can provide you automatic customizable research density calculations of any unit and type, along with tools and visualizations to explore and better understand your institution’s research enterprise.
Want to Learn More?
For the next post in this series, we’ll discuss the difference between gross and net investment in research as well as why and where each matter. In the meantime, if you’re interested in learning more or seeing what a research density product would look like for your institution, please contact us here or reach out to the authors directly via linked emails below. As creators of Attain Apps, a SaaS product line focused on academic and research institutions, we are passionate about enterprise performance and metrics and always enjoy sharing, learning, and collaborating with customers.
About the Authors
Sander Altman
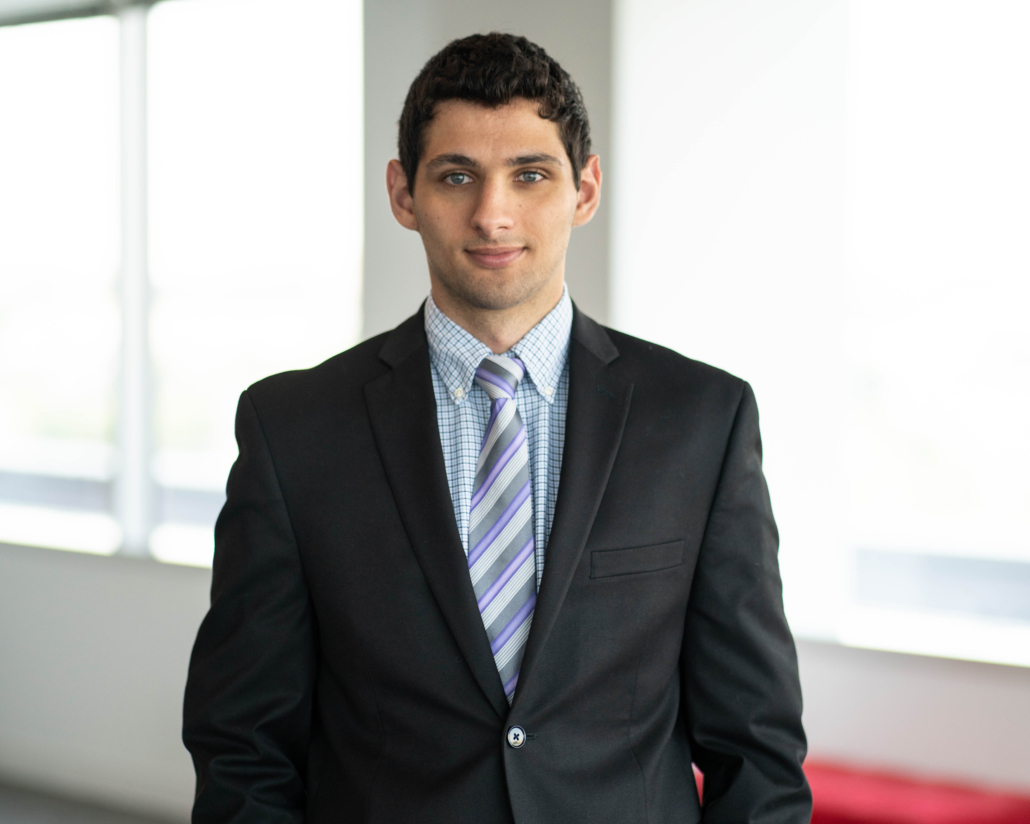
Sander Altman is the Chief Architect for the Product and Innovation business at Attain Partners. As the technical leader behind Attain Apps since its formation in 2017, Sander has extensive experience with the technology empowering the platform, as well as a developed understanding of the subject matter covered by the various products within the platform. With a background in AI and Intelligent Systems, as well as an M.S. and a B.S. in Computer Science, Sander has focused on providing institutions with intelligent and easy-to-use software to optimize their academic and research enterprises.
Alexander Brown
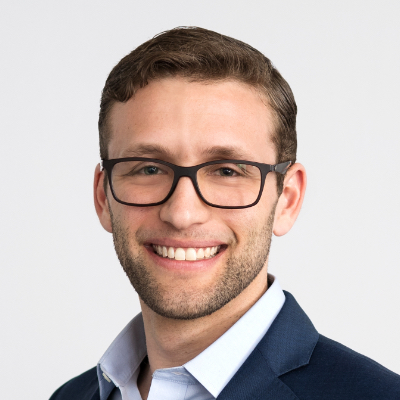
Alexander Brown is the Practice Leader for the Product and Innovation business at Attain Partners. Alex is responsible for the full product lifecycle of the Attain Apps product line, which features the firm’s cornerstone intellectual properties distilled into easy-to-use SaaS products. With a background in economics, and experience as a designer, developer, and consultant himself, Alex works hand-in-hand with experts and developers to create products that provide academic and research institutions with best practices and insights in an affordable and convenient package.